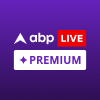
OpenAI Unveils New Tool, CriticGPT, To Find Errors In AI-Generated Code: Report
In a study, CriticGPT demonstrated competence in analysing code and identifying errors that may elude human notice, aiding in the detection of hallucinations.
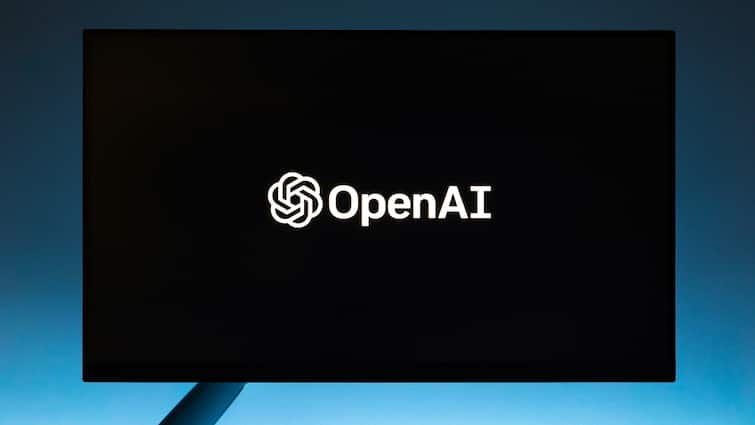
OpenAI has reportedly designed a new AI model based on ChatGPT-4, CriticGPT. This newly designed AI model will help in identifying the users' errors in code produced by ChatGPT. According to reports, this new AI model is in the trials and it has already improved code review outcomes by a whopping 60 per cent. OpenAI is likely to include CriticGPT into OpenAI's Reinforcement Learning from Human Feedback (RLHF) labeling pipeline. It is expected that the company aims to provide AI trainers with more efficient tools to evaluate complex AI outputs.
The GPT-4 models powering ChatGPT aim to enhance interactivity and utility through RLHF (Response Learning from Human Feedback). This involves AI trainers evaluating and rating various responses to improve their quality. As ChatGPT's reasoning abilities advance, errors are becoming more subtle, posing challenges for trainers in identifying inaccuracies.
In a study titled 'LLM Critics Aid in Detecting LLM Errors,' CriticGPT demonstrated competence in analysing code and identifying errors that may elude human notice, aiding in the detection of hallucinations. The researchers trained CriticGPT on a dataset containing intentionally inserted bugs in code samples, enabling it to recognise and flag coding errors effectively.
ALSO READ | Detective Dotson Xbox Release Announced By Masala Games — Check Details
What’s More To Come?
Reportedly during experiments of CriticGPT, teams using CriticGPT gave more holistic critiques and identified fewer false positives when compared to the ones who were working alone. LLM Critics Help Catch LLM Bugs reported, “A second trainer preferred the critiques from the Human+CriticGPT team over those from an unassisted reviewer more than 60 per cent of the time, as reported by.”
Critics have raised concerns about CriticGPT's capabilities, noting that it appears to have been trained primarily on brief responses from ChatGPT. This suggests a need for further development to effectively handle longer and more complex tasks. Additionally, a significant challenge that remains is the phenomenon known as 'ChatGPT hallucinating,' where the AI model generates incorrect information and presents it as factual, which CriticGPT has yet to fully address.
Moreover, there are occasional labelling errors made by trainers, and a notable limitation lies in the focus on isolated errors rather than addressing issues that span multiple aspects of a response. This limitation is closely linked with RLHF. As these advanced models become increasingly knowledgeable, there is concern that human trainers may find it challenging to provide meaningful feedback effectively within the CriticGPT framework.
Top Headlines
Trending News
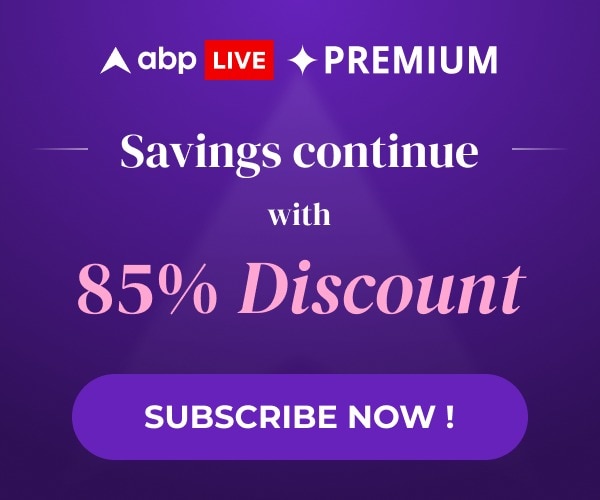
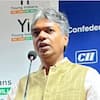